Our Editors-in-Chief’s Top Picks
The Editors-in-Chief of our CASS publications have selected some noteworthy papers from the recent issues of our journals:
IEEE Transactions on Circuits and Systems II: Express Briefs
Paper 1:
B. Saux, J. Borgmans, J. Raman and P. Rombouts, "Origin of Frequency-Dependent Distortion and Calibration for Ring Oscillator VCO ADCs," IEEE Transactions on Circuits and Systems II: Express Briefs, vol. 71, no. 8, pp. 3670-3674, Aug. 2024, doi: 10.1109/TCSII.2024.3370121. https://ieeexplore.ieee.org/document/10445510
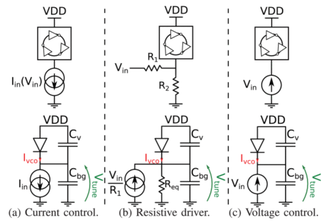
Summary: This work identifies the cause of frequency-dependent distortion in ring oscillator voltage-controlled oscillator (VCO) analog-to-digital converters (ADCs). First, a VCO model is presented which takes into account the capacitance at the VCO terminals. This allows the most common drive configurations to be analyzed to determine the origin of the frequency-dependent distortion and the vulnerability of the different drive configurations.
Paper 2:
X. Lu et al., "A 0.013 mm² 3.2-ns Input Range 10-Bit Cyclic Time-to-Digital Converter Using Gated Ring Oscillator With Phase Domain Reset in 65-nm CMOS," IEEE Transactions on Circuits and Systems II: Express Briefs, vol. 71, no. 8, pp. 3635-3639, Aug. 2024, doi: 10.1109/TCSII.2024.3367177. https://ieeexplore.ieee.org/document/10439976
Summary: This work presents a compact gated ring oscillator (GRO) based cyclic time-to-digital converter (TDC) for single-photon emission computed tomography, 3D cameras, and fluorescence lifetime imaging microscopy. The proposed cyclic TDC also features a phase domain reset scheme which generates a compensation pulse for purging the GRO phase residue among consecutive conversion cycles. The TDC achieves a high resolution of 3.1ps together with an extended input range of 3.2ns, with 1.41mW at 36MS/s from a 1-V supply.
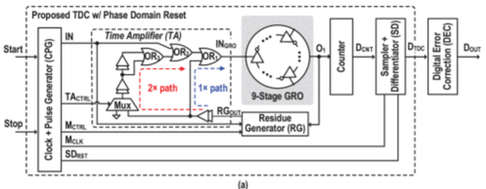
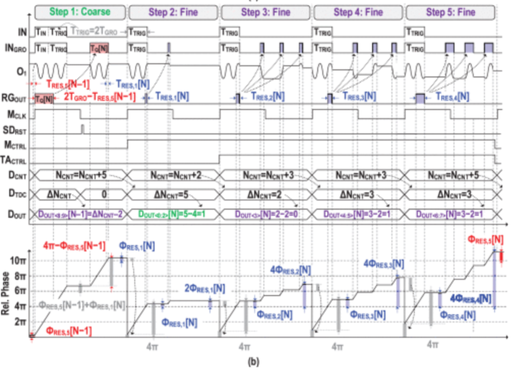
Paper 3:
P. Kaesser, J. Wagner, O. Ismail and M. Ortmanns, "Inner Transfer Functions in Incremental ΔΣ ADCs," in IEEE Transactions on Circuits and Systems II: Express Briefs, vol. 71, no. 9, pp. 4111-4115, Sept. 2024, doi: 10.1109/TCSII.2024.3390397. https://ieeexplore.ieee.org/document/10504533
Summary: In this brief the transfer functions from inner nodes of incremental Delta-Sigma (I-DS) analog-to-digital converters (ADCs) are analyzed. Such transfer functions are needed in order to predict the attenuation of error sources like noise and distortion from within the loopfilter (LF).
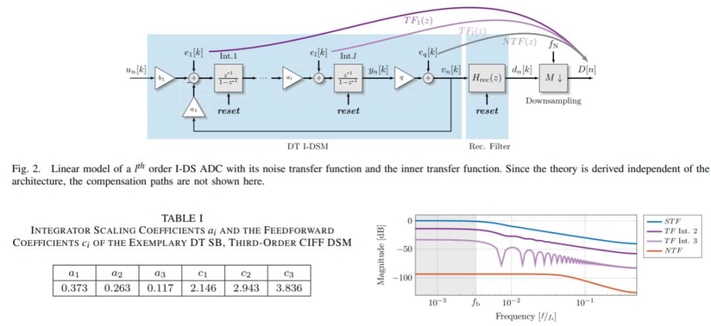
IEEE Transactions on Circuits and Systems for Video Technologies
Paper 1:
W. Wen et al., "Perceptual Quality Assessment of Virtual Reality Videos in the Wild," IEEE Transactions on Circuits and Systems for Video Technology, vol. 34, no. 9, pp. 8368-8381, Sept. 2024, doi: 10.1109/TCSVT.2024.3378352. https://ieeexplore.ieee.org/document/10473760
Summary: In VR-related applications, investigating how people perceive virtual reality (VR) videos in the wild (i.e., those captured by everyday users) is a crucial and challenging task due to complex authentic distortions localized in space and time. There are some existing panoramic video databases, but they only consider synthetic distortions, assume fixed viewing conditions, and are limited in size. In this paper, authors aim to overcome these shortcomings and constructed the VR Video Quality in the Wild (VRVQW) dataset, containing 502 user-generated videos with diverse content and distortion characteristics. The collected VRVQW dataset in then used to conduct a formal psychophysical experiment to record the scanpaths and perceived quality scores from 139 participants under two different viewing conditions. A thorough statistical analysis of the recorded data is provided observing significant impact of viewing conditions on both human scanpaths and perceived quality. Authors also developed an objective quality assessment model for VR videos based on pseudocylindrical representation and convolution. Results on the VRVQW show that the method is superior to existing video quality assessment models. The dataset and the code are available at https://github.com/limuhit/VR-Video-Quality-in-the-Wild
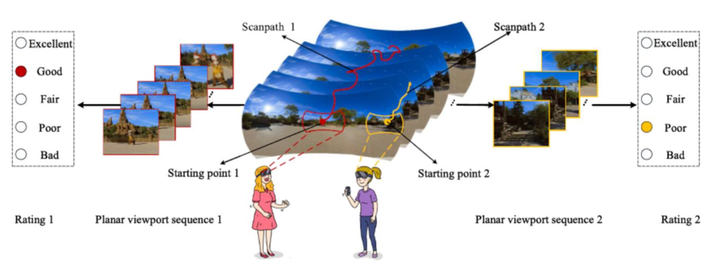
The above Illustration shows how people explore VR videos in the VRVQW dataset as proposed in this work. Under varying viewing conditions (e.g., starting points and exploration times), users may exhibit different viewing behaviors in the form of scanpaths, leading to different portions of the video being explored. As user-generated VR videos often come with localized authentic distortions, the perceived quality may vary with user viewing behaviors constrained by viewing conditions. Therefore, the incorporation of viewing conditions would be the key to the success of computational quality prediction of user-generated VR videos.
Paper 2:
F. Wan, X. Miao, H. Duan, J. Deng, R. Gao and Y. Long, "Sentinel-Guided Zero-Shot Learning: A Collaborative Paradigm Without Real Data Exposure," IEEE Transactions on Circuits and Systems for Video Technology, vol. 34, no. 9, pp. 8067-8079, Sept. 2024, doi: 10.1109/TCSVT.2024.3384756. https://ieeexplore.ieee.org/document/10489996
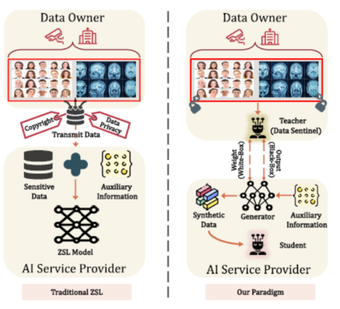
Summary: This work proposes an innovative Sentinel-Guided Zero-Shot Learning (SG-ZSL) paradigm that aims to reduce concerns over data privacy and model copyrights, especially in the context of collaborations between AI service providers and data owners. The proposed SG-ZSL is designed to foster efficient collaboration without the need to exchange models or sensitive data. It consists of a teacher model, a student model and a generator that links both model entities. The teacher model serves as a sentinel on behalf of the data owner, replacing real data, to guide the student model at the AI service provider’s end during training. Considering the disparity of knowledge space between the teacher and the student, two variants of the teacher model have been introduced: the so called omniscient and the quasi-omniscient teachers. Under these teachers’ guidance, the student model seeks to match the teacher model’s performance and explores domains that the teacher has not covered. To trade-off between privacy and performance, two distinct security level training protocols have been introduced: white-box and black-box, enhancing the paradigm’s adaptability. Despite the inherent challenges of real data absence in the SG-ZSL paradigm, this model outperforms in ZSL and GZSL tasks, notably in the white-box protocol. A comprehensive evaluation further attests the robustness and efficiency of the method across various setups, including stringent black-box training protocol.
In traditional ZSL approaches, real data is necessitated to establish the visual-semantic association. The proposed SG-ZSL approach, instead, introduces a teacher model, which acts as a data sentinel, enabling the execution of ZSL tasks without the need for direct access to real data.
Paper 3:
Y. Yuan et al., "Learning Discriminative Features via Multi-Hierarchical Mutual Information for Unsupervised Point Cloud Registration," IEEE Transactions on Circuits and Systems for Video Technology, vol. 34, no. 9, pp. 8343-8354, Sept. 2024, doi: 10.1109/TCSVT.2024.3379220. https://ieeexplore.ieee.org/document/10475373
Summary: Extracting discriminative representations is the key step for correspondence-free point cloud registration. The extracted representations require to be discriminative to transformation, which demands representations to reduce the influence of redundant information irrelevant to transformation. However, recently proposed methods ignore this crucial property, resulting in limited ability to represent point cloud. This paper aims to relieve features redundancy issues for correspondence-free point cloud registration from a new perspective. In particular, the proposed method comprises two stages: a feature extraction stage and a rigid body transformation stage. In the feature extraction stage, the objective is that of maximizing multi-hierarchical mutual information between different hierarchical features; this can provide discriminative and less redundancy representations to regress transformation parameters for the next stage. In the rigid body transformation stage, dual quaternions are used to estimate transformation parameters, which combines rotation and translation simultaneously within a unified framework and obtains a compact representation for rigid transformation. The proposed model is trained in an unsupervised manner on the ModelNet40 dataset. The experimental results show that the method proposed in this work achieves higher accuracy and robustness compared with existing correspondence-free methods.
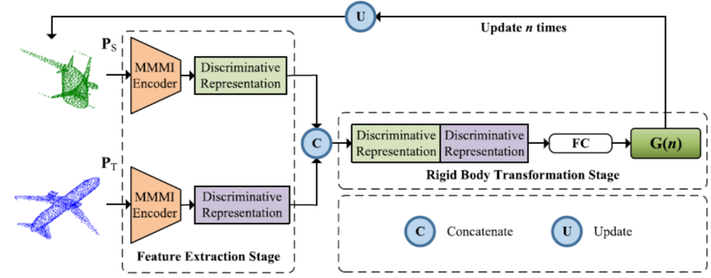
The method proposed in this paper is divided into two stages: the feature extraction stage and the rigid body transformation stage. In the feature extraction stage, a novel structure is proposed to reduce features redundancy by Maximizing Multi-hierarchical Mutual Information (MMMI) between hierarchical features, which can obtain discriminative representation. Then, two discriminative representations are concatenated. In the rigid body transformation stage, dual quaternions are utilized to represent transformation by regression. In order to achieve more precise transformation, a source point cloud updating strategy is employed to refine the transformation.
IEEE Journal on Emerging and Selected Topics in Circuits and Systems
Paper 1:
P. Du, Y. Liu and N. Ling, "CGVC-T: Contextual Generative Video Compression With Transformers," IEEE Journal on Emerging and Selected Topics in Circuits and Systems, vol. 14, no. 2, pp. 209-223, June 2024
https://ieeexplore.ieee.org/document/10496072
Paper 2:
H. Sun, Q. Yi and M. Fujita, "FPGA Codec System of Learned Image Compression With Algorithm-Architecture Co-Optimization," IEEE Journal on Emerging and Selected Topics in Circuits and Systems, vol. 14, no. 2, pp. 334-347, June 2024
https://ieeexplore.ieee.org/document/10494759
Paper 3:
Y. Zeng et al., "Physically Guided Generative Adversarial Network for Holographic 3D Content Generation From Multi-View Light Field," IEEE Journal on Emerging and Selected Topics in Circuits and Systems, vol. 14, no. 2, pp. 286-298, June 2024
https://ieeexplore.ieee.org/document/10495040
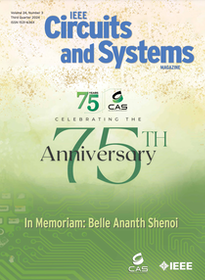
________________
IEEE CAS Magazine Third Quarter Issue 2024
Features
A Compact Electronically Tunable Meminductor
Emulator Model and Its Application
Pankaj Kumar Sharma, Rajeev Kumar Ranjan, and Sung-Mo Kang
Chiplet-GAN: Chiplet-Based Accelerator
Design for Scalable Generative
Adversarial Network Inference
Yuechen Chen, Ahmed Louri, Fabrizio Lombardi, and Shanshan Liu
________________
IEEE JETCAS Call for Papers: Generative AI Compute: Algorithms, Architectures, and Applications to CAS
Scope and Purpose
Generative Artificial Intelligence (GenAI) is one of the most heated and emerging topics. Both academia and industry are actively embracing GenAI for its astounding capabilities and wide range of applications. Towards its full implementation and ultimate prosperity, the assistance of the circuits and systems (CAS) community plays a pivotal role. However, the increasing scale of GenAI models poses unprecedented challenges to their practical deployment, creating a huge gap between GenAI and the field of circuits and systems. The challenges faced by contemporary GenAI implementations are multifaceted:
- Resource limitations: Circuits and systems, which are limited by fixed computational resources, find it challenging to keep pace with the relentless expansion of GenAI models.
- Architectural rigidity: The one-size-fits-all endeavor of existing hardware architectures fails to cater to the diverse, personalized requirements that different GenAI applications necessitate.
- Energy constraints: The escalating computational demands of GenAI, growing virtually at an exponential rate, cannot be met sustainably by energy-constrained circuits and systems.
Addressing these challenges calls for joint efforts from algorithm, implementation, and design perspectives. First, algorithm optimizations towards efficient GenAI deployment is essential. Researchers are actively exploring complexity reduction techniques to streamline generative models without significantly compromising their performance. Though recent algorithm research has made progress on pruning and quantization, such downsized GenAI models remain resource-intensive. Thus, there is an urgent need for hardware-aware GenAI algorithms, while keeping the superior performance. Second, efficient circuits and systems for GenAI are imperatively needed. Innovative hardware and architectures for GenAI are continuously being proposed, aiming to strike a balance among scalability, flexibility, and efficiency. Companies in industry are making strides, but there is a continuous need for specialized GenAI accelerators and energy-efficient computing paradigms for GenAI. Third, GenAI for accelerating circuits and systems design is of great need and promising. GenAI also has the potential to enhance electronic design automation tools, emulate circuits, optimize simulations, and accelerate verification. However, challenges remain in ensuring reliability, efficiency, and trust.
The objective of this special issue is to solicit and present the latest research findings in the field of circuits and systems for GenAI, and GenAI-aided design for circuits and systems.
Topics of Interest:
To give a comprehensive introduction to this field, we plan to solicit papers presenting the latest developments in algorithms, implementations, and applications related to GenAI, as well as GenAI-aided design for circuits and systems. Within the broad scope, we prioritize the following areas of interest:
- Algorithm optimizations for efficient GenAI deployment. This special issue is interested in algorithm optimizations for efficient GenAI deployment, particularly optimizing algorithms with respect to hardware compatibility, and presenting low-complexity, hardware-friendly models for emerging applications, e.g., image generation, language translation, and genomic research.
- Circuits and Systems for efficient GenAI implementation. This special issue invites papers on designing circuits and systems for efficient GenAI implementation, including architecture design for GenAI, circuit design for ASIC/FPGA, and embedded software implementation on multi-core CPU/GPU/DSP. This special issue also welcomes papers that address implementation-related issues, such as architecture security, performance evaluation and comparison, and scalability.
- GenAI for accelerating circuits and systems design. This special issue also highlights how AI can transform the design methodology of circuits and systems. This can be applied to design automation, efficient verification, and other related cases. Examples may include large language models (LLMs) and other GenAI for ASIC/FPGA circuits.
Given the multidisciplinary flavor of this special issue, we welcome papers with topics in any of the following areas and beyond:
- Computational complexity reduction of GenAI
- Resource-efficient frameworks for GenAI workloads
- Hardware-friendly optimizations of GenAI
- Software/hardware co-design of GenAI
- Innovative key-value caching strategies of GenAI
- Ethical and security considerations in the deployment of GenAI
- Efficient architectures of GenAI
- Hardware accelerator design for GenAI
- Resource-efficient implementations of GenAI
- Energy efficient implementations of GenAI
- Memory optimizations for GenAI implementations
- In-memory computing architectures for GenAI
- Implementation of GenAI in various fields
- Frameworks for secure and scalable implementation of GenAI
- GenAI and circuits and systems in emerging applications
- GenAI-aided very large-scale integrated circuits design
- LLM-aided circuits and systems design
- Neural networks for customized circuits and systems
Submission procedure:
Prospective authors are invited to submit their papers following the instructions provided on the IEEE JETCAS website: https://ieee-cas.org/publication/JETCAS/manuscript-submission-guide. The submitted manuscripts should not have been previously published, nor should they be currently under consideration for publication elsewhere. The IEEE JETCAS submission site: https://ieee.atyponrex.com/journal/jetcas
Important dates
- 2 December 2024: Manuscript submissions due
- 20 January 2025: First round of reviews completed
- 3 March 2025: Revised manuscripts due
- 31 March 2025: Second round of reviews completed
- 14 April 2025: Final manuscripts due
Request for information
Corresponding Guest Editor: Chuan Zhang (chzhang@seu.edu.cn)
Guest Editors:
Chuan Zhang
Southeast University, China
Email: chzhang@seu.edu.cn
Naigang Wang,
IBM T.J. Watson Research Center, USA
Email: nwang@us.ibm.com
Jongsun Park
Korea University, Korea
Email: jongsun@korea.ac.kr
Li Zhang
University of Leeds, UK
Email: l.x.zhang@leeds.ac.uk
________________
IEEE JETCAS Call for Papers: 2.5D/3D Chiplet Circuits and Systems, EDA, Advanced Packaging, and Test
Scope and Purpose
The task of integrating an increasing number of transistors onto a single chip is becoming increasingly arduous and costly. To counter this challenge, chiplet technology has captured the interest of both industry and academia. A chiplet is a small integrated circuit (IC) with a well-defined function, designed to be incorporated alongside other chiplets within a single package as a multi-die stack. Despite the current interest in a chiplet-based design approach, the associated technology faces numerous challenges that are influencing its development trajectory. This special issue is dedicated to showcasing the latest technological advancements in the field of chiplet technology and its applications.
In general, the chiplet interconnects faces design challenges such as achieving high bandwidth, low latency, high energy efficiency, high edge bandwidth density, low bit error rate, etc. There are existing standards for chiplet with no consideration on compatibility with each other at PHY layer specification, this raises the implementation cost of IP vendors and SoC designers.
Designing a chip based on the chiplet approach presents the challenge of how to effectively split a monolithic design into individual chiplets. An improper division will negatively impact the chip's performance and power efficiency. Furthermore, SoC designers must carefully consider the design of the system bus or I/O connected by chiplet interconnects, and keep the underlying structure remains transparent to the upper-layer software.
When developing a chip based on the chiplet approach, the SoC designer will meet the lack of comprehensive EDA tool coverage from front-end to back-end. For example, there are no EDA tools for multi-chiplets co-simulation in the front-end phase. They must leverage existing EDA tools that were initially designed for the development of monolithic SoC designs. Although multi-physics field co-simulation is utilized to identify potential risks before the tape-out phase, it is more beneficial and cost-effective to detect and mitigate issues during the front-end phase.
While chiplets are typically considered to be connected by interposers in advanced packaging, new methods such as silicon bridges have garnered public attention due to their lower cost. Hybrid bonding is also emerging as a promising technique for its extremely high density. 3D stacked ICs based on the chiplet approach draw more attention for their efficient way to integrate functional chiplets, but also result in more problems such as thermal.
2.5D- and 3D-stacked ICs based on the chiplet approach have many more potential test moments than conventional chips. Every test that is executed adds cost, and therefore, executing all these tests might lead to overkill and excessive test costs. However, not executing tests could lead to even higher costs. At the same time, pre-bond testing of the non-bottom dies is a challenging task, and we need to rely on a cooperative design-for-test infrastructure in the die-under-test and all dies below it.
The objective of this special issue is to give a systematic view of chiplet technology from many aspects, to find possible solutions for challenges, and to compile the latest research findings from researchers in the field.
Topics of Interest
To give a comprehensive introduction to this field, we are soliciting paper submissions presenting the latest developments in SoC architecture for chiplet-based IC and chiplets, chiplet interconnects, dedicated EDA tools along the entire design trajectory, 2.5D/3D advanced packaging, 2.5D/3D test and DfT. This special issue’s area of interest includes (but is not limited to) the following topics:
- Architecture exploration for chiplet-cased ICs
- System and technology co-optimization (STCO) for chiplet and advanced packaging
- System bus design in chiplet-based ICs
- General purpose computing chiplet
- Accelerator chiplet
- High-speed and low power I/O chiplet
- Multi-protocol combination design in I/O chiplet
- High-bandwidth memory chiplets
- High-speed design for chiplet interconnects
- Energy-efficient design for chiplet interconnects
- Multi-standard support in chiplet interconnects
- Adaptable I/O design for chiplet interconnects
- Floorplanning, partitioning, placement and routing optimization for chiplet-based IC by AI/ML
- Interconnect planning and synthesis
- Package/3D-IC placement and routing
- Extraction, TSV, and package modeling
- Deterministic/statistical timing analysis and optimization for chiplet-based IC
- Multi-chiplet functional co-simulation
- Multi-physics field co-simulation
- 2.5D/3D packaging and platforms
- Si/Glass/Organic-based interposer
- Si/Glass/Organic-based RDL
- Fanout wafer level and panel level packaging
- Hybrid bonding
- New chiplet and advanced packaging concepts and platforms
- Chiplet and stack description languages for advanced packaging
- SI and PI design for chiplet and advanced packaging
- Thermal design and materials in chiplet and advanced packaging
- Electromagnetic compatibility design in chiplet and advanced packaging
- Yield and cost estimation of stacked dies for chiplet and advanced packaging
- Stress, warpage, and reliability issue for chiplet and advanced packaging
- Built-In self-test (and repair) for chiplet interconnects
- Defects in chiplet Interconnects
- Design-for-test for and repair of chiplet interconnects
- DfT architectures for chiplet-based ICs
- EDA design-to-test flow for chiplet interconnects
- Failure analysis for chiplet interconnects
- Fault-tolerant design for chiplet interconnects
- Interposer testing
- Pre-, mid- and post-bond testing
- Reliability of chiplet interconnects
- Standards for chiplet interconnect test and repair, incl. IEEE Std P3405
- Standards for chiplet testing, incl. IEEE Std 1838
- Test flow optimization for chiplet interconnects
- Test pattern generation for chiplet interconnects
- Yield of stacked dies and their Interconnects
Submission procedure:
Prospective authors are invited to submit their papers following the instructions provided on the IEEE JETCAS website: https://ieee-cas.org/publication/JETCAS/manuscript-submission-guide. The submitted manuscripts should not have been previously published, nor should they be currently under consideration for publication elsewhere.
The IEEE JETCAS submission site is https://ieee.atyponrex.com/journal/jetcas.
Important dates
- 3 March 2025: Manuscript submissions due
- 21 April 2025: First round of reviews completed
- 2 June 2025: Revised manuscripts due
- 30 June 2025: Second round of reviews completed
- 21 July 2025: Final manuscripts due
Request for information
Corresponding Guest Editor: Qinfen Hao (haoqinfen@ict.ac.cn)
Guest Editors:
Qinfen Hao
Chinese Academy of Sciences
(haoqinfen@ict.ac.cn)
Kuan-Neng Chen
National Yang Ming Chiao Tung University
(knchen@nycu.edu.tw)
Sandeep Kumar Goel
TSMC
(skgoel@tsmc.com)
Hai Li
Duke University
(hai.li@duke.edu)
Erik Jan Marinissen
imec
(Erik.Jan.Marinissen@imec.be)
______________________________
Latest Tables of Contents of CAS Sponsored Journals
The latest issues of our CAS sponored journals have been published and the tables of contents can be accessed through the following links:
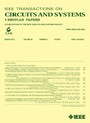
- IEEE Transactions on Circuits and Systems I: Regular Papers
- IEEE Transactions on Circuits and Systems II: Express Briefs
- IEEE Transactions on Circuits and Systems for Video Technology
- IEEE Journal on Emerging and Selected Topics in Circuits and Systems
- IEEE Circuits and Systems Magazine
- IEEE Transactions on Biomedical Circuits and Systems
- IEEE Design and Test Magaz